Visibility into AI Agents
AI agents could pose novel risks. Information about their activity in the real world could help us manage these risks.
Alan Chan
This blog post summarises the paper “Visibility into AI Agents,” by Alan Chan and a multi-institution team of co-authors. The paper is forthcoming at FAccT 2024.
GovAI research blog posts represent the views of their authors, rather than the views of the organisation.
Introduction
Researchers are, increasingly, working to develop AI agents: AI systems that can pursue real-world goals with minimal supervision.
Arguably, rudimentary AI agents already exist. For example, ChatGPT is no longer a simple question-and-answer chatbot. It can now perform tasks such as searching for information on the internet, writing and running computer code, and creating calendar events.
Future agents may be capable of performing much longer and more complex sequences of tasks by themselves. They may eventually be assigned many important, open-ended responsibilities.
In part because they need less human steering and oversight, advanced AI agents could pose novel risks. One foundational concern is that people will not have enough visibility into their behaviour. For example, users of AI agents may not always know if their agents are misbehaving.
Therefore, we should make sure that key stakeholders will have enough visibility into AI agents. Obtaining visibility will mean thoughtfully collecting, processing, and sharing information about what agents are doing.
This post explores three particular measures that deployers — the companies that run AI agents for users — can take to create helpful forms of visibility. These measures are:
- Agent identifiers that allow third parties to know when they are interacting with an AI agent
- Real-time monitoring that allows deployers to notice agent misbehaviour, including misbehaviour that a user might not have noticed on their own
- Activity logs that allow deployers or users to review an agent’s past behaviour, to notice and better understand problems
Some of these measures are already in use for certain AI systems. In these cases, we discuss how to adapt them for agents.
More work is needed to understand how and when to implement these measures, since they also raise significant concerns around privacy and the abuse of power. Still, if implemented well, visibility measures could improve our understanding of AI agents, help reduce accidents and misuse, and generate data to inform governance decisions.
AI agents and their risks
AI agents are AI systems that can pursue goals in the world under limited supervision. Current AI agents are still fairly rudimentary: they can only carry out certain short and simple tasks, such as creating calendar events and doing basic web searches. They struggle to perform many specific tasks, chain tasks together, and plan their actions.1
However, researchers are working to create AI agents that can perform far longer and more complex sequences of tasks with minimal human supervision.2 An advanced AI agent might operate more like a high-autonomy employee — who is given a high-level goal, pursues it with minimal oversight, and only occasionally reports back — and less like a simple tool. It could take on a range of personal, commercial, or even governmental responsibilities from people. These kinds of advanced AI agents could be very useful.
At the same, AI agents could also pose significant risks. For example, AI agents may pose greater misuse risks than more tool-like AI systems, because they have broader capabilities and rely less on the skill of human users. AI agents may also have a greater potential to cause harm if they malfunction or suffer from controllability issues.3 New risks could also emerge from interactions between different AI agents, similar to the kinds of risks that we have already seen emerge from interactions between high-frequency trading bots.
Any risks from AI agents will be exacerbated because — by default — people will not have as much visibility into their behaviour: agents would accomplish tasks under limited supervision. Someone using an AI agent could therefore have only limited knowledge and understanding of what their agent is doing. Similarly, when an AI agent interacts with a third party, this third party might not even know they are interacting with an AI agent. In such cases, risks created by AI agents may remain unnoticed or poorly understood for long periods of time.
Hence, it will be especially important to ensure that key stakeholders have enough visibility into the behaviour of AI agents. This information could directly help deployers and users avoid accidents and instances of misuse. It could also offer insight into emerging risks and thereby inform governance decisions and safety research.4
Obtaining visibility into the use of agents
We consider three ways to obtain visibility into the use of agents: agent identifiers, real-time monitoring, and activity logs. These measures could provide governments and civil society the information necessary to plan for and respond to the deployment of more advanced agents.
We focus on how deployers—companies like OpenAI and Anthropic that run agents for users—could implement visibility measures. Deployers are a useful intermediary because they tend to have access to all of an agent’s inputs and outputs, which facilitates implementation of visibility measures.5
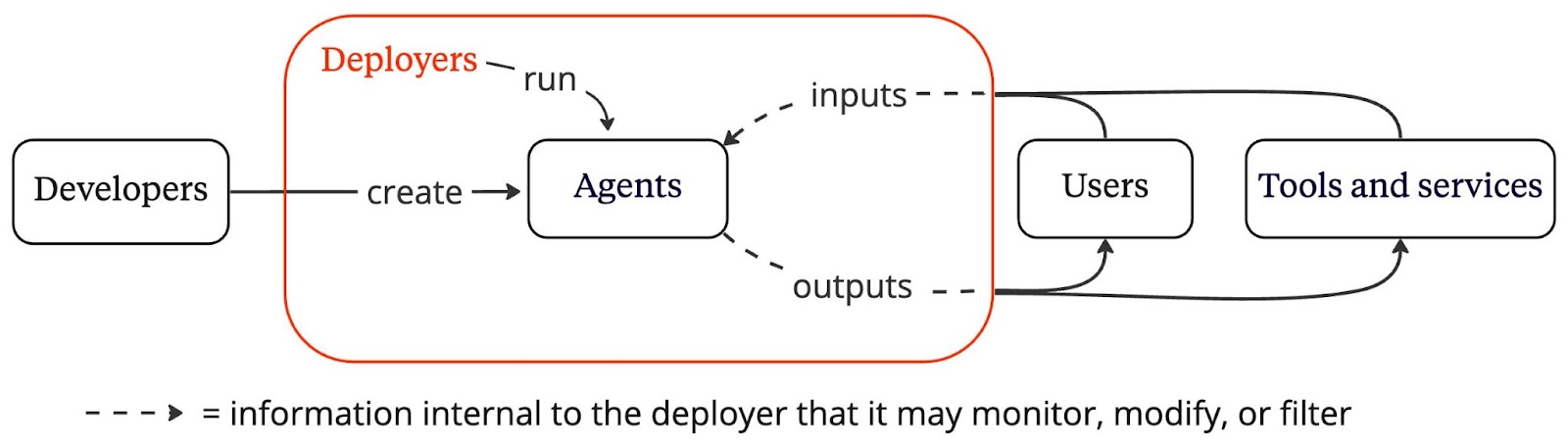
First, an agent identifier is an indicator that would allow a person or software program to identify when they are interacting with an agent. Examples of agent identifiers include automated chatbot disclosures or watermarks, but many other kinds of agent identifiers could be created. For instance, when an agent performs a financial transaction with a bank, the deployer could send a unique ID associated with the agent to the bank. Additional information about the agent could be attached to the identifier as well, such as whether the agent has satisfied certain security standards.
The potential usefulness of AI agents means that they may be involved in many consequential interactions. Since agents would carry out activities with little to no human supervision, parties to an interaction would have the option to change how they interact with the agent. For instance, parties could refuse such interaction, or may take additional precautions to check the agent’s outputs. As such, agent identifiers could help participants in an interaction decide when and how to interact with AI agents. Widespread use of agents across a variety of consequential tasks may also be of interest to regulators. Aggregated information about agent identifiers could help measure the overall level of AI agent activity across various domains.

Second, real-time monitoring would involve oversight of agent activity in real time, whether by humans or software. Just as social media companies filter or flag abusive content, deployers could do the same for agent behaviours. Indeed, since agents may take consequential actions without users knowing, real-time monitoring may be especially important for ensuring that users approve the actions.
Some deployers already monitor model use for abuse, but monitoring protocols will need to be adapted for the wider variety of actions that agents could perform. For example, agents could carry out a variety of financial transactions on their users’ behalf. To reduce the risk of harmful or unintentional actions, financial transactions above a certain dollar amount could be flagged for user review. Moreover, monitoring protocols that focus upon a single system may be insufficient. If groups of agents can cause harmful feedback loops or other sources of instability, monitoring would need to account for their collective activities.
One limitation of real-time monitoring is that it is most effective when a human overseer or other computer program can swiftly assess a flagged behaviour and determine an appropriate course of action. Consequently, monitoring may be most useful in cases where the flagged behaviour clearly violates established policies or guidelines.

Third, activity logs could record some of an agent’s inputs and outputs for further analysis. Logs are helpful for understanding the risks of agents because harmful behaviour may only be recognisable as such long after its occurrence.6 Users or deployers reviewing logs may notice that an agent has taken a consequential action—which real-time monitoring might not have caught—without approval. As such, logs could inform real-time monitoring, help investigate incidents, and track long-run impacts of agent usage.
Although logging is a common practice for software systems, agent-specific logging practices could be especially useful. Logs dedicated to certain parts of an agent could illuminate factors that affect an agent’s impact in the real-world. For example, memory or reasoning logs could help us understand why an agent malfunctioned in the pursuit of a complex, long-horizon goal. Logs about an agent’s use of tools or services could help us identify the impacts of an agent on third parties. It may also be important to analyse the logs of multiple agents so as to understand risks that come about from their interactions.
Overall, visibility measures like agent identifiers, real-time monitoring, and activity logs could help track the impact of AI agents by collecting data on their activities. These data could help to inform potential interventions to prevent and respond to malfunctions.
Limitations and risks of obtaining visibility
While these visibility measures have the potential to substantially improve our understanding of AI agents, they also have some limitations and pose risks of their own.
First, we have thus far only discussed visibility measures for deployers. However, users can also run AI agents themselves using cloud computing resources or their own hardware. In these cases, the burden would ultimately be on users to ensure that the agents they are running are providing appropriate identifiers. These users would also be responsible for running the real-time monitoring and activity-logging software that is needed to detect misbehaviour. However, some users may not choose to take these precautions. Self-monitoring also does not prevent cases of intentional misuse.
One alternative option in this case may be to have providers of cloud compute services implement variants of visibility measures. For example, compute providers could track especially large uses of compute and investigate whether they correspond to potentially concerning uses of agents. Additionally, providers of external tools could require proof that agents accessing their tools have implemented visibility measures. For example, a bank could require a digital signature that uniquely identifies the agent accessing its services.7
On the whole, though, obtaining visibility when the deployment of AI agents is “decentralised” is much more difficult. When deployers no longer have access to an agent’s inputs and outputs, it is harder to ensure that relevant information about the agent will be shared.
Second, whether or not visibility measures involve deployers, they raise significant concerns regarding privacy and abuse of power. Information about the actions of AI agents may reveal significant information about their users. Those collecting such information could abuse their power, for example to engage in excessive surveillance or the inappropriate sale of personal data. Valuable services could be barred to AI agents that did not come from approved deployers. If so, users may be locked into using agents that do not serve their best interests or meet their specific needs.
An important question is therefore how to design data collection and access practices that both provide useful information about agents and minimise the misuse of the information. One approach may be to limit visibility measures to high-stakes domains. For example, visibility measures might be a higher priority for agents that interact with the financial or legal systems. Another option may be to limit the granularity of collected information. In some cases, it may suffice to know that an agent has simply accessed a certain tool without knowing the precise inputs and outputs.
Finally, information is insufficient for reducing risks. We also need effective processes to allow relevant actors to use and act on the information. Once AI agents and visibility measures are widely deployed, they will likely generate a lot of data. In light of the rapid pace of AI progress, delays in acting on this information could be quite costly.
Conclusion
Given the potential impact of AI agents, visibility into their use is important for understanding and limiting risks. Identifying agents in their real-world interactions, monitoring their behaviour in real time, and keeping records of their behaviour could all be effective strategies for ensuring safety.
However, more work is required to understand how, when, and to what extent these visibility measures could be best implemented. In addition to addressing potential misuse of the information generated from visibility measures, we also need efficient processes for making use of the information to inform actions. Ideally, visibility could guide the use of a wide range of strategies by individual users, deployers, and governments to manage the risks of AI agents.
The author of this piece would like to thank the following people for helpful comments on this work: Markus Anderljung, Stephen Clare, and Ben Garfinkel.
Alan Chan can be contacted at alan.chan@governance.ai
Footnotes
1 - See GAIA: a benchmark for General AI Assistants, AgentBench: Evaluating LLMs as Agents, Large Language Models Still Can't Plan (A Benchmark for LLMs on Planning and Reasoning about Change) | OpenReview
2 - As one example of ongoing progress, see this recent work to create an AI software engineer.
3 - These risks could be compounded if it is difficult or costly to turn some agents off, even once their malfunctioning is noticed. This might occur if they become widely integrated into societal systems.
For example, consider AI agents that are heavily integrated into the financial system. If we come to depend upon the speed and expertise of such agents for a large number of financial transactions, human alternatives for all those transactions may be infeasible. Just as we deem certain financial institutions “too big to fail,” so too may certain AI agents become too essential to shut off.
4 - Although pre-deployment assessments (including model evaluations) are useful, they cannot completely substitute for post-deployment visibility measures. It is unlikely that a developer will be able to identify and resolve all safety issues before an AI system is deployed. For example, new safety issues can emerge when users augment AI systems with new tools.
5 - Although they may not actively take advantage of this access because of, e.g., privacy concerns.
6 - As a potential example, consider the diffuse effects of social media.
7 - For more discussion on how to identify when an AI agent is accessing a service rather than a human, see Section 4 of our paper.